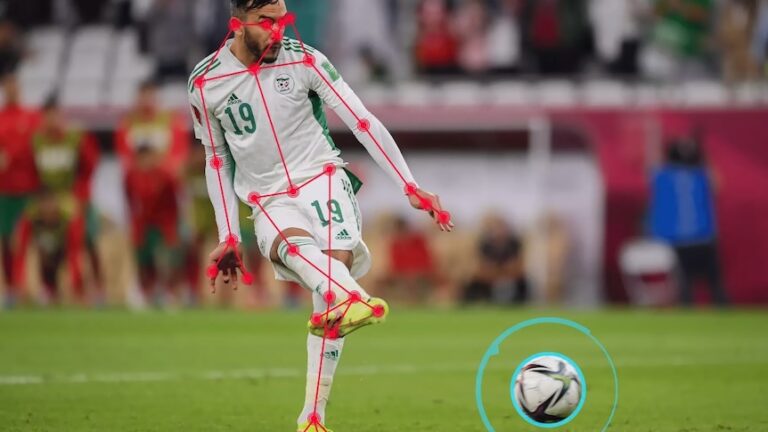
If you’ve watched any of the televised World Cup matches, you’ll have glimpsed the new technology being used by the VAR (video assistant referee) to help determine when a player is offside.
Though it usually appears on the TV screen for just a few seconds every match, it’s arguably one of the stars of Qatar 2022.
Semi-automated offside technology (SAOT) has taken decades to develop, requires sophisticated artificial intelligence (AI) systems, a battery of special cameras, as well as a ball packed with sensors, and about 10 years ago would have seemed impossible.
The computer vision technology it relies on is powering a new generation of data gleaning services, which are changing the way “the world game” and other sports are being coached and played.
Tracking every limb of every player
To refresh your memory, here’s how SAOT appears on TV: When a potential off-side is detected, play is stopped, and the view abruptly shifts to a virtual representation of the match, with the players appearing as mannequin-like figures in their player jerseys, frozen mid-stride.
A plane of translucent white running across the width of the field shows the exact position of the offside line.
If a single inch of an attacker (apart from their hands and arms) is beyond this line, they’re offside (pending the referee’s decision).
This may sound straightforward, but when you stop to think about what’s required to make this system both possible and reliable enough to use in a World Cup, it raises all kinds of questions.
Like, how does SAOT know the location of not only every player on the pitch, but the position of their limbs, down to the millimetre?
The story of how this technology was developed intersects with a motley assortment of other stories, from the real-life events behind the movie Moneyball, to England getting knocked out of the 2010 World Cup, to the origin of hugely popular Snapchat filters.
It begins in the summer of 1966.
A summer project that lasted decades
Computer vision, or teaching a computer to visually recognise objects, is something that may sound easy, but is really, really hard.
Take, for example, the task of recognising a bird. A computer can be shown a photo and taught that a particular pattern of pixels adds up to an object called “bird”. But birds flap around. They hop and move. The pattern of pixels changes with every photo.
To teach a computer to recognise a bird, you have to teach it to interpret what those pixels represent. You have to somehow teach it to recognise the common “bird” within millions of photos.
For some reason, at first it was thought this would be easy.
In 1966, MIT computer scientist Marvin Minsky assigned a first-year undergraduate student this problem to solve over the summer.
Needless to say, the student didn’t solve it, although their work laid the foundations for the field, says Simon Lucey, director of the Australian Institute for Machine Learning at the University of Adelaide.
“A lot of people thought that it would be really simple to get machines to sort of see like we as humans do,” he says.
“But it’s turned out to be obviously extremely difficult.”
The following decades saw slow progress. Robots could be taught to recognise boxes on assembly lines, for instance, or to read hand-written postcodes on envelopes, but that was about it.
Then, in 2012, there was a sudden advance.
“2012 was a big inflection point, and the inflection point was these things called deep neural networks,” Professor Lucey says.
“[They] basically allowed computer vision to jump from this sort of theoretical curiosity where governments were funding things … to actually companies realising that, ‘Hey, we can use this stuff.'”
The dawn of cat filters
From this 2012 inflection point flowed the computer vision applications that we use every day, like iPhone Face ID and Google reverse image search, as well as systems that are used on us, from facial recognition surveillance to police cars that scan number plates.
But first: What is a deep neural network (DNN)? Neural networks are designed to imitate how humans think and learn; they’re made up of layers of nodes, much like the human brain is made up of neurons. The network is said to be deeper based on the number of layers it has.
To teach a computer to recognise a bird in photos, a DNN can be “trained” to do this by feeding it a dataset of images that contain birds and those that do not, with each image labelled “bird” or “not bird”.
Through analysing these images and isolating the elements that make up the object that the DNN has been tasked with learning (beak, wings, feathers), the machine learns to “see” birds.
This idea isn’t new, but the advent of more powerful computers and the availability of huge amounts of data for training the networks has made them significantly more capable.
That’s exactly what happened in 2012, when a DNN called AlexNet smashed its rivals at the annual ImageNet computer vision competition.
“Typically, when technology improves, there’s sort of a creep; it moves along slowly every year,” Professor Lucey says.
“Here, in 2012, there was this massive improvement and the entire world went, ‘what’s this?”‘
Three years later, the first Snapchat filters came out. Using the AlexNet approach, they worked by mapping the features of a user’s face, then adding an artistic overlay, such as an animal’s ears and nose.
From Moneyball to automated player tracking
But it wasn’t only useful for adding whiskers to a human face. Among those taking notice was the company Hawk-Eye, which at the time was best known for providing automated line calls at the tennis, or in plotting the projected path of the ball in leg-before-wicket decisions at the cricket.
Although useful and impressive, the ball-tracking technology didn’t require any advanced computer vision.
“It was developed in the ’90s because we didn’t have to have advanced AI to be able to track a yellow tennis ball,” Professor Lucey says.
But the AlexNet breakthrough opened other possibilities for the application of computer vision in sport, and it came at a time when FIFA, football’s world governing body, was looking for ways to finally remove persistent human error from refereeing decisions.
A catalyst for change was when England was knocked out of the 2010 World Cup after losing to Germany, in a match where an England goal that should have been given was controversially disallowed.
FIFA approved the use of Hawk-Eye goal-line technology two years later.
Also taking notice of improvements in computer vision were sports data and analytics companies.
Over the previous decade, professional sport had undergone a quiet revolution in data analytics, with managers, recruiters and coaches increasingly hungry for reams of esoteric player and match data that would decode the secrets of the game, and give their team the edge.
A frequently cited example of this was the success of the Oakland Athletics in the 2002 baseball season (later told in the 2011 movie Moneyball), after they utilised computer-generated analysis to buy new players.
“Really, sports analytics starts with Moneyball,” says Patrick Lucey, chief scientist at the sports and data analytics company Stats Perform.
“Instead of using human intuition, they used data-driven decisions.”
Broadcasters also were wanting data to spice up their coverage, (like “heat maps” tracking player movement), while betting agencies were demanding data-driven predictive analyses.
But there was a problem: this data had to be collected manually, which was incredibly arduous.
“Humans were watching the game and they would basically do physical measurements, just basically seeing how far they’ve run, and how many sprints and jogs,” Dr Lucey says.
“They would log where [the player] was at each frame.”
Through the noughties, a hidden army of humans were logging each frame of hundreds of games and recording such minutiae as when a pass or shot occurred, or who stepped where.
By 2013, automated systems had arrived. Stats Perform was using computer vision to track balls and players in every match of the NBA.
Opta, the sports data subsidiary of Stats Perform, is now the world’s largest provider of data for soccer, including for Football Australia. You’ve almost certainly seen their data being used in broadcast coverage.
“[Automated] player tracking has been used in soccer for over a decade now,” Dr Lucey says.
“You can measure pass options at every frame. You measure the likelihood of, ‘Should I pass to this player, should I pass to that player. What’s the likelihood of us creating a chance in the next 10 seconds?’
“Through this measurement tool and then machine learning on top, we can start to measure things that you couldn’t before.”
Computer says no
The evolution of the technology has gone from tracking balls (eg for LBW decisions), to tracking players, and, finally, at this year’s World Cup, to tracking the limbs of the players.
Semi-automated offside technology (SAOT) made its debut at the 2021 Arab Cup, but has been mostly kept under wraps until Qatar 2022.
It works much the same way as automated player tracking: Twelve cameras fixed in positions around the stadium track 29 points on each player’s body 50 times per second.
“It’s not so much the cameras, it’s more the technology behind it,” says Robert Aughey, a sports scientist at Melbourne’s Victoria University.
“It’s the way the images are turned into the skeletal-tracking modelling. It’s definitely challenging to do — and do accurately.”
Victoria University is an official FIFA research institute and Professor Aughey has been testing the accuracy of SAOT, as well as other semi-automated refereeing systems.
Cameras aren’t the only components of the system, he says. Because offside is determined at the moment a player is passed the ball, the ball used at World Cup matches contains a sensor with an accelerometer and gyroscope to measure the exact moment it’s kicked.
“If you’re relying on a video with 50 frames per second, sometimes you can miss the exact kick point. It can happen between frames,” he says.
This level of accuracy has resulted in players being judged offside by margins not visible in the stadium, or on the video screen.
“I’m going to have a blooming high blood pressure by the end of the month if this carries on this way,” former England striker Alan Shearer told the BBC, after a goal by Ecuador was disallowed for offside.
“I don’t think there’s any person watching this in the world who thinks that this is offside.”
Professor Aughey’s response to this is: Get used to being wrong.
“It’s just an example of where the technology can be more accurate than what the naked eye can see.
“I think FIFA is pretty happy with [SAOT] to be fair. I wouldn’t be surprised if the Premier League rolls it out for next season.”
Where to from here?
What’s significant about SAOT isn’t just that it works, but that FIFA trusts it will work every time, says Professor Lucey from Adelaide University.
“This is a great example of where AI has traditionally struggled — high-risk scenarios.
“You’ve got billions of people around the world watching this, and they’re relying on it to be correct. If it’s incorrect, you’re going to have a lot of upset countries.”
Dr Lucey at Stats Perform says the next step will be “scale” — rolling out automated player tracking, and the data insights this allows, to lower leagues; everything from junior club soccer to college basketball.
“Now you can measure what you couldn’t measure before — what was an intuition.
“Sports data is really about reconstructing the story of the match. The more granular the data, the better the story that you can tell.”
Loading
Some may not like the idea that the mysteries of football, or any sport, can be reduced to figures on a spreadsheet. Or the notion that anyone with a tablet of player data can be as good as a seasoned coach may strike them as insulting.
But, fortunately, that’s not what Dr Lucey is saying.
He says some metrics can’t be measured. Not all variables can be calculated.
“We’re good when we can digitise the data, but there are some things that we can’t digitise.
“We don’t know what’s been said [to the players]. We don’t know the emotions there. You’re not sure who’s been reading social media. We’re not sure who’s had a fight with their wife. We’re not sure whose child’s been sick.”
Ahead of the World Cup, Opta predicted who would win. And it was wrong.
Its predicted winner, Brazil, was knocked out in the quarter-finals by Croatia.
Opta also predicted that Morocco would come bottom of its group, but instead the “Atlas Lions” finished on top, and then beat favoured teams like Spain and Portugal to make it all the way to the semi-finals.
As computer vision peers into the heart of professional sport, there are some things it cannot see, Dr Lucey says.
“It’s very hard to measure randomness.
“Having these automatic methods can give us a pretty good indication, but then that’s where the human takes over.”